Introduction
A/B testing is one of the most powerful tools in a marketer’s toolbox. It helps you optimize conversion rates and better understand your audience’s behavior. But while the idea of “test and learn” seems simple on paper, reality is much trickier. Without a clear hypothesis, with small sample sizes, or when tests are rushed or poorly executed, the insights you gather can be misleading — or even harmful.
The result? Instead of improving your website or campaign performance, you end up hurting your conversions and making decisions based on faulty data. Below, you’ll find 25 common A/B testing mistakes that marketers make — along with practical advice on how to avoid them and ensure your tests actually help, not harm, your strategy.
How to Start A/B Testing Without Slipping Up
One of the most common mistakes is starting without a clear hypothesis. Running a test “just to see what happens” leads to chaotic data and confusion. You need to define what you're testing, why you're testing it, and what outcome you expect.
Another pitfall is not segmenting your audience. Lumping all users into one group may hide important behavioral differences. For instance, mobile vs. desktop users or new vs. returning visitors often react very differently. Without segmentation, you risk making decisions based on averaged data that doesn't reflect reality.
Many marketers also test the wrong pages. It’s easy to spend time optimizing your “About” page — but unless it plays a key role in conversion, your efforts may be wasted. Focus instead on high-impact pages, like product pages, checkout flows, or lead generation forms.
Running a test without enough traffic is another critical error. If your sample size is too small, you won’t reach statistical significance, and your results will be unreliable — or flat-out wrong. And while A/B tests are designed to isolate variables, don’t forget that user behavior is interconnected. A change shown to one segment can indirectly influence others through social sharing, referrals, or word of mouth.
Lastly, avoid running your tests in a silo. If your A/B testing isn’t aligned with other departments — like sales, content, or paid ads — you might miss out on important insights or create inconsistencies across your marketing channels.
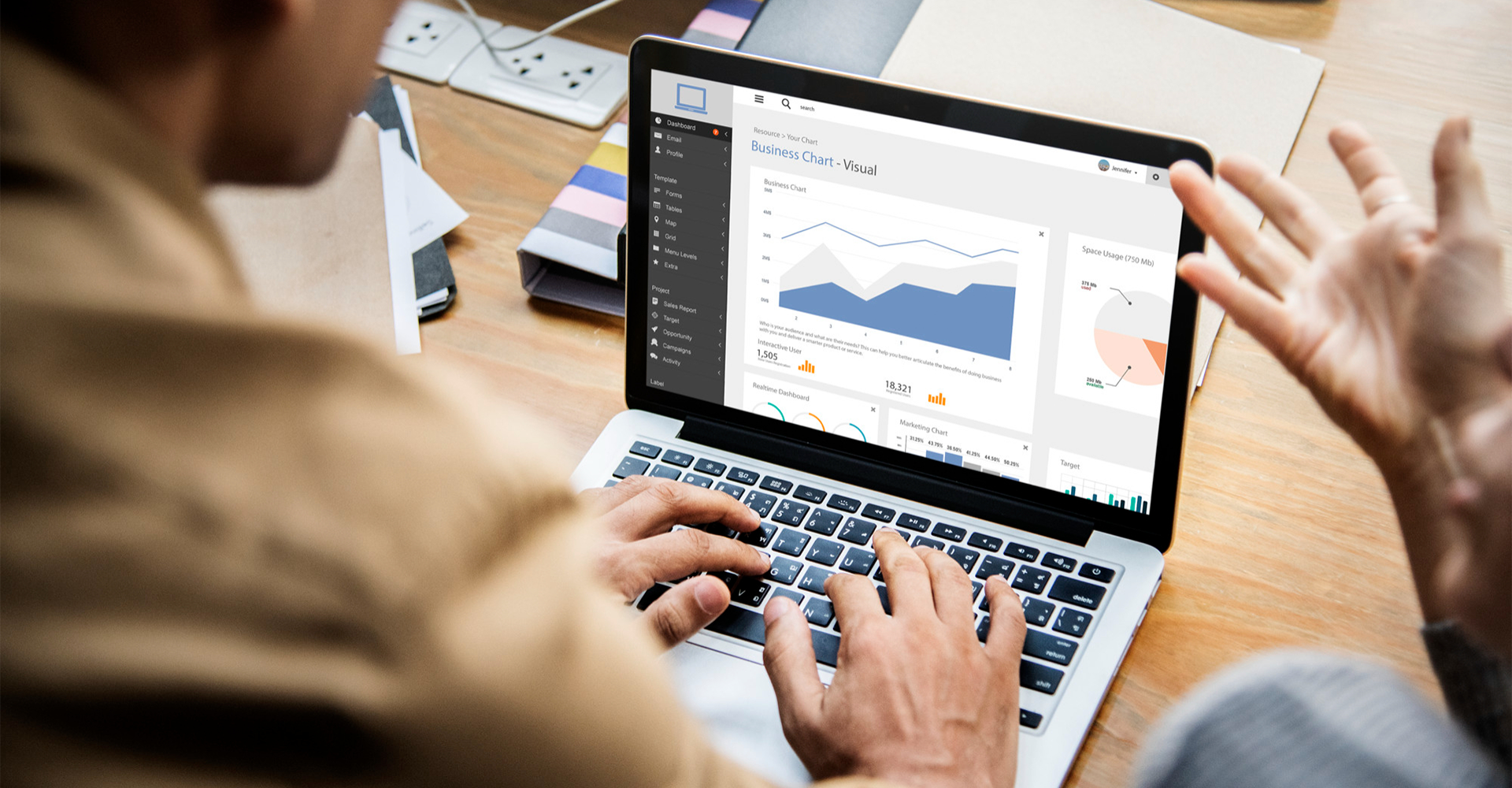
What Can Go Wrong During an A/B Test
During testing, one classic mistake is prioritizing design over functionality. A beautiful layout might look impressive, but if it confuses users or obscures key actions, you’re hurting performance. Likewise, just because testimonials work for one company doesn’t mean they’ll work for yours — or for every audience segment. Always test assumptions before treating them as truth.
Another major risk is losing brand voice. Optimization shouldn’t come at the cost of your identity. If your variant feels disconnected from the tone or values of your brand, users may feel confused or mistrustful.
One of the most damaging mistakes is ending the test too early. Everyone wants fast results, but stopping a test before it has matured leads to unreliable conclusions. It’s also important to ensure your testing tool is fast and optimized — slow-loading pages increase bounce rates and skew results.
Avoid running multiple tests at once on the same audience unless you’re using multivariate testing methods. Mixing signals makes it nearly impossible to know which change drove which result. Also, be careful not to compare data across different timeframes (e.g., weekdays vs. weekends), and never modify test variables mid-experiment, as this completely invalidates the results.
Post-Test Pitfalls That Undermine Your Efforts
After a test is complete, don’t forget to document everything — your hypothesis, audience, timeframe, results, and insights. This creates a library of knowledge your entire team can build on. Don’t be discouraged if a test “fails” — an underperforming variant still provides valuable insights. Often, it’s the first step toward discovering what actually works.
Resist the urge to overhaul your site all at once just because one variant performed better. Implement changes gradually and continue observing user behavior. Also, make sure you’re measuring the right metrics. Misconfigured tools or goals (e.g., broken Google Analytics events) can lead you astray.
Never fall into the trap of blindly copying another brand’s case study. What worked for them may not work for you — different industries, user intents, and customer journeys demand unique approaches. Equally important: don’t ignore small wins. A 2–3% improvement might seem modest, but over time, those small gains compound into major growth.
Make sure you have a strategic testing roadmap, not just a series of isolated experiments. A chaotic approach results in wasted resources and missed learnings. You should also understand validity threats, like a variation not displaying properly on certain devices or browsers. Technical bugs can corrupt your data and mislead your analysis.
Don’t assume your results are universally applicable. A test that performs well on desktop may flop on mobile. If you don’t segment post-test results, you risk applying the wrong lesson to the wrong group. Also, be aware of downstream effects — an improvement in click-through rates (CTR) might lead to worse outcomes later in the funnel (e.g., lower time on site or higher bounce rates).
And remember: a tie isn’t a failure. If both versions perform similarly, you’ve still learned something important — neither change made a big enough difference, and it may be time to try a new hypothesis or test a different area of your funnel.
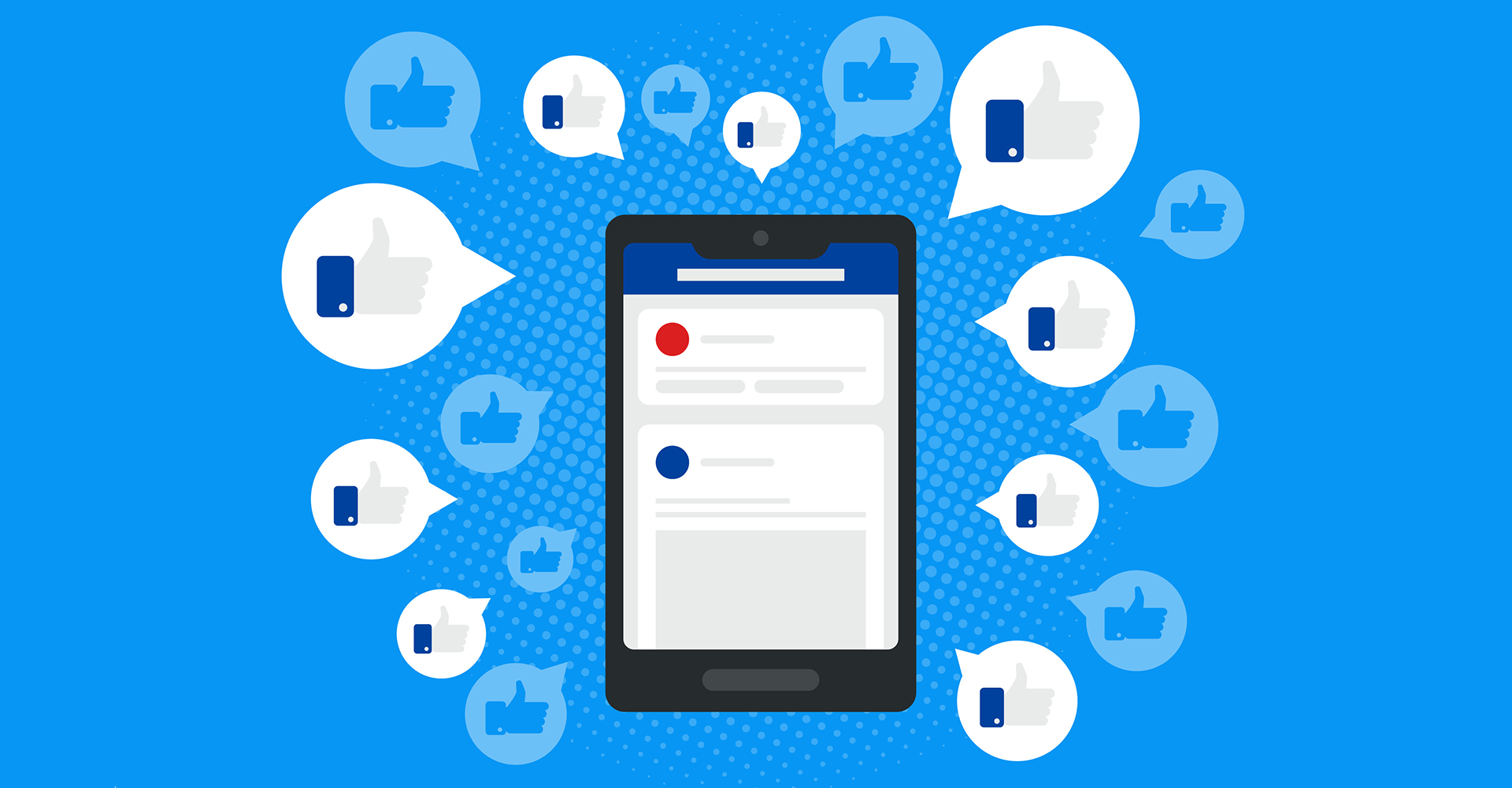
Conclusion
A/B testing offers an incredible opportunity to continually improve user experience and business performance — but only when executed carefully. Each of the 25 mistakes above has the potential to distort your findings and lead you in the wrong direction.
Focus on clear hypotheses, proper audience segmentation, statistically valid sample sizes, and reliable analytics. Track your results, analyze them deeply, and most importantly — be patient and methodical.
Even “no change” is valuable insight, showing you what’s already working or highlighting where you can look for innovation. And when you’re ready to test more effectively and efficiently, consider using platforms like Unbounce, which offer built-in A/B testing tools and real-time optimization features.